First In-Person Conference (RLDM 2022) at Brown University!
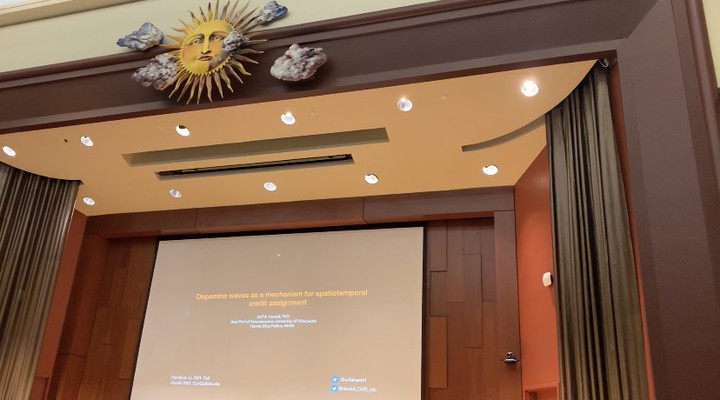
First time being in Providence and in an in-person conference for RLDM 2022, it was an inspiring experience to mingle with a number of researchers from various fields including psychology, neuroscience, and computer science.
The conference lasted for four days, and it started with two introductory and two advanced tutorials. The following three days consisted of invited talks, contributed talks, poster sessions and workshops. The dynamics of speakers, who have been invited or selected to the conference under the same research realm called reinforcement learning (RL), helped integrate broad, diverse, and inconsistent views on the definitions, goals, and implications of RL. In the advanced tutorial by Dr. Xiaoxi Gu from Mount Sinai Hospital, the subjective utility of an agent was defined as the summation of self’s utility and other’s utility in a certain decision-making scheme (e.g., auction). As a clinical psychology researcher, it was fascinating to learn about broad implications of this approach in understanding psychiatric disorders such as nicotine addiction. Dr. Oriel HeldmanHall from Brown University presented interesting works done by Joesph Heffner that in social paradigms, mood valence prediction error quantified in a 500 * 500 grid was the strongest predictor of social decisions even after accounting for reward prediction error. The arguments that self’s decision-making may be governed by other’s utility or mood valence beyond reward prediction error opened up two questions that whether the social and affective components should be incorporated into the RL algorithms utilized in computer science and whether they will significantly moderate actions in less social environments.
In a perspective of a psychologist, the talks from the computer science areas were focusing mainly on maximizing cumulative reward. Some speakers questioned what ‘reward’ should be, but most intuitively it was something that an agent tries to gain, not loss or avoid. The agent is told what the reward is and learns the function (policy) to maximize cumulative reward by, for instance, minimizing a loss function. Also, the agent can successfully ‘discover’ the goal by forming a ‘question’ itself, which was presented by Dr. Satinder Singh Baveja from DeepMind and University of Michigan. RL in Robotics focuses on replicating human behaviors such as locating a place, performing sequential actions (e.g., finding a carrot and chopping it), or distinguishing sounds from different objects. These actions also follow the main goal of maximizing cumulative reward as the robot’s actions and their underlying algorithms are adjusted based on accuracy measures - if the robot finds the exact location only two out of ten attempts exploiting a current policy, it will update the policy to minimize the failed attempts and to reach higher hit rates. Even though these RL algorithms lead to successful reward maximization, these goals and policies did not necessarily account for the influences of other agents and emotions. There might be some RL frameworks accounting for cooperative behavior, but it is hard to find any aspects of mood being included in the non-human agent RL models.
Due to the complicated and volatile nature of the affective components, such trend is totally understandable. It has not been easy to investigate how moods vary and affect learning even in human beings. Any learning agents can be told or can form the question (“what to achieve?") in a given state, and it is expected that they wwould decide upon the policies consistent with the question. However, human actions can be swayed by momentary moods. Even if we know the exact goal, we sometimes choose the actions against the goal because we are feeling too positive or too negative. This example might imply the role of mood extremeness on decision-making, but it is just one of the potential hypotheses.
I’m not sure if it is advantageous to take moods into account when modeling a RL agent in computer science because moods can lead to non-reward maximizing choices. One thing that this conference has taught me though is a need to incorporate these affective components into clinical RL studies for better understanding mood disorders like depression and anxiety. Even in the environments that do not involve others and are less social, individuals with depression or anxiety often face situations that their decisions are influenced by their mood states, leading to a vicious cycle of psychiatric symptoms. These moods, however, might not be captured well within current lab-based decision-making tasks, because of the moods' contextual or even voluntary representations over choices. I would like to clarify the ‘contextual and voluntary’ representations in another blog post to keep the main theme in this post.
Among many factors affecting decision-making and learning in mood disorders, I believe mood-related factors are crucial. Through this conference, my research goals have been reinforced to delve more into the roles of moods and emotions in less social but clinically relevant states. For one final remark, there is a therapeutic technique called validation. Validation is often achieved by accepting naturally occurring emotions. Basically helping clients feel and recognize that it is very natural for them to feel such emotions in the given situations. When serving as a clinician, this acceptance by the clients themselves seemed to be one of the most important keys for alleviating depressive and anxious symptoms. This abstract concept of validation might be better understood through applying computational modeling to empirical studies. One possible way would be developing a computational model to tract the degree to which individuals with depression can validate their feelings during a decision-making task and to study how validation is associated with their choices. This sounds vague for now, but excited to further develop this idea as one of my long-term research initiatives :)